Glossary
Data Transformation
Data transformation involves transitioning data from an external source to an internal information system.
In the era of Big Data, organizations possess vast volumes of data, which they can use to help them make better decisions. However, not all data is immediately usable. Before it can be used, it is essential to ensure its quality and standardize its format. This is precisely the role of data transformation.
What is Data Transformation?
Data transformation is the process of converting data from one format to another to maintain consistency across all of an organization’s data. Data often comes from a diverse range of sources (social media, CSV files, CRM, relational databases, websites, etc.). It is often created or provided in a raw format, which is unusable and/or incompatible with other data.
The idea behind data transformation is to ensure compatibility by harmonizing all internally used data.
Once transformed, the data is ready to be integrated into the company’s information system, stored in a data lake or data warehouse, analyzed, and leveraged to create value.
Why Transform Data?
Data transformation offers numerous benefits to organizations. Here are some examples:
- Data Integration: The primary goal of data transformation is to facilitate data enrichment, allowing information from various sources to be combined successfully.
- Decision-Making: Following data integration, companies can make better-informed decisions by identifying patterns and extracting meaningful insights from multiple data sources.
- Data Sharing: Data transformation makes data more comprehensible and accessible, facilitating internal sharing among teams or departments, as well as external sharing with partners or clients. This helps break down data silos.
- Data Reuse: Data sharing also promotes data reuse by third parties, enabling the creation of new services and adding more value through data.
What Are the Steps in Data Transformation?
Data transformation seamlessly integrates into ELT (extract load transform) or ETL (extract transform load) processes. While both models are essential for effective data management, the ETL process is favored in modern data management.
The first step involves collecting data from various sources. Data transformation can then begin, following these steps:
- Discovery: Once data is collected, it must be understood. In some cases, a preliminary cleaning phase is necessary to eliminate duplicates, or false, obsolete, or inconsistent data.
- Mapping: This stage involves planning the data transformation.
- Filtering: Relevant data is filtered based on predefined parameters.
- Code Generation and Execution: Given the exponential volume of data, code generation facilitates data transformation. Once generated, the code is executed, and the data is converted according to the predefined format.
- Validation: Ensures that the data has been correctly formatted.
Which Tools are used for Data Transformation?
It is possible to use separate software tools for each step listed above, for instance, a data profiling tool for the discovery phase, a mapping solution, coding tools, and so on.
However, the simplest approach is to use an ETL solution that manages all the stages of data transformation. These tools often provide parallelization, monitoring, failover, coding, automation, and more. Additionally, they often generate visual representations of data flows, making it easier to understand the transformation process.
Comprehensive ETL solutions greatly support data reuse by centralizing and providing transformed information to everyone.
Learn more
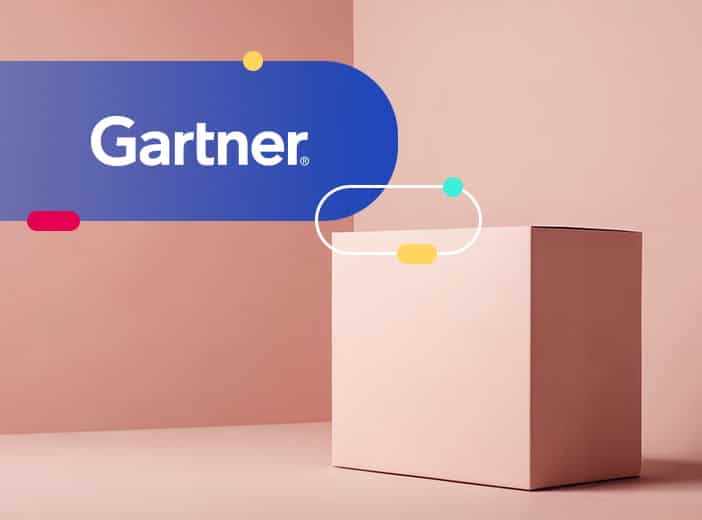
Delivering long-term data product success – lessons from Gartner
How can you create and scale data product programs? Based on new insights from Gartner, we explain the key processes required in building relevant data products that meet user needs on an ongoing basis, emphasizing the importance of data product marketplaces to drive consumption and ROI.
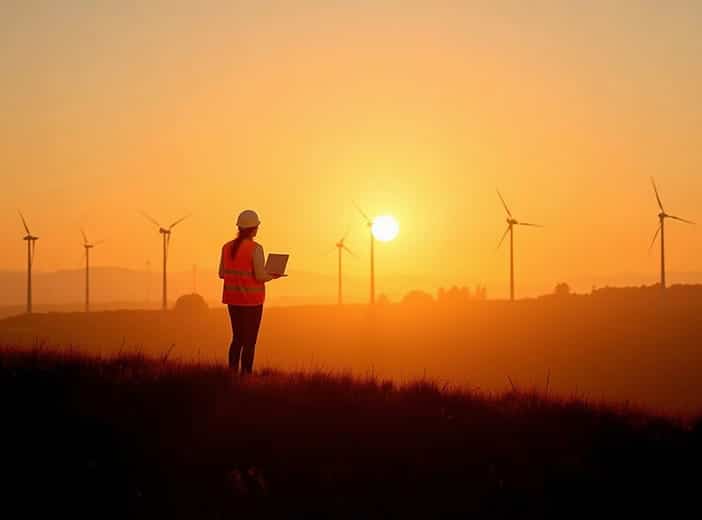
How energy data marketplaces drive ROI and financial benefits
Data sharing through data marketplaces is essential to deliver the collaboration and management required to build decarbonized energy ecosystems. But the benefits extend much further - we explore the financial ROI effective data sharing delivers.
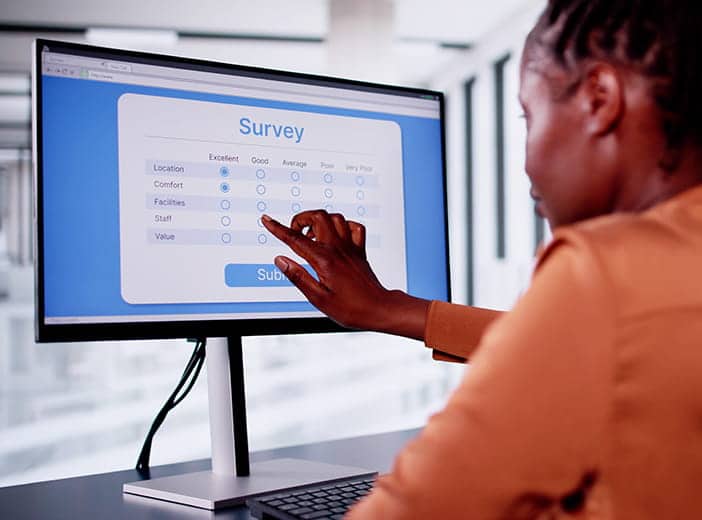
Increasing data collection and driving collaboration through built-in forms
Data doesn’t just come from business systems and data producers - it can equally be provided by users and data consumers, widening the range of assets on your data marketplace. We explain how collecting data through integrated forms increases engagement, democratizes collection and strengthens your data community.