What are the benefits to using your data portal to feed AI models?
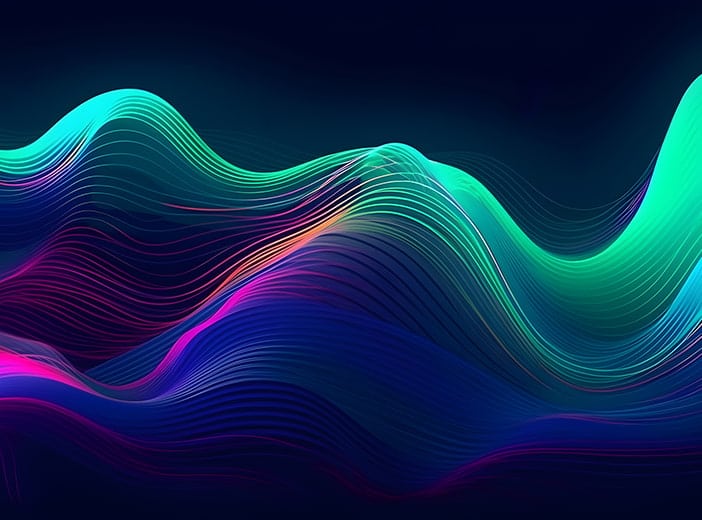
Learn how data portals enhance the training and effectiveness of artificial intelligence models by providing reliable, high-quality and trustworthy data, which is essential to ethically deploy AI and harness its benefits.
Whatever industry you operate in, understanding and harnessing artificial intelligence (AI) is now seen as critical to business success. Whether in the finance, healthcare, technology, manufacturing or utility sectors, AI is transforming processes, improving performance and paving the way for unprecedented innovation.
Used intelligently and with the right safeguards in place, artificial intelligence represents an extraordinary opportunity to change how organizations operate and accelerate growth. However, its effectiveness depends completely on the quality of the data that feeds it. The old saying, “garbage in, garbage out” has never been so true. In fact, out of date and unreliable data might compromise the usefulness of artificial intelligence in the long term.
Data portals are the obvious solution to this problem, delivering the data that in turn provides the foundation for trusted and effective AI models. This article explains how to bring AI and data portals together to drive AI success.
AI models: how are they created and are they trustworthy?
Artificial intelligence models are primarily based on machine learning (ML) and deep learning (DL). These are trained on large datasets, learning to recognize patterns, make predictions, translate, transcribe or generate content, in the case of generative AI. The quality of their outputs depends directly on the quality of this training data. Biased, incomplete or out of date information can lead to errors and impact the performance of services developed on the basis of these AIs. And because the underlying algorithms are not publicly available, errors can be difficult to spot before it is too late.
Well-publicized issues include:
- Microsoft’s Tay chatbot and Facebook’s algorithm: when the former was provoked by users to create racist comments on social networks, the latter then created recommendations that further spread this content.
- Google Ads: Based on its algorithm, it was shown to advertise high-paying jobs more to men than to women. Amazon, via an internal recruitment AI, experienced a similar issue.
- OpenAI’s ChatGPT: Although powerful, until recently ChatGPT 3.5 could only produce answers based on outdated information, as it was trained on data that stopped in 2021.
The evidence is clear: when an algorithm is trained on simplified data or contains the cognitive biases of its designer, the quality of its output suffers. This impacts its usefulness and reliability. To combat these problems, AI teams responsible for designing algorithms need to be aware of their biases, and use datasets that are representative and high-quality to avoid any unwitting distortions when training algorithms.
Essential criteria to providing AI models with trusted datasets
To guarantee the reliability of artificial intelligence, it is essential that the data used meets three main criteria:
- Reliability: Firstly, the data must be accurate and exclude any bias that could compromise its veracity. In addition, regular data updates are crucial to ensure the relevance of models in constantly evolving environments. Obsolete data could lead to errors in predictions or decision-making. The data used to train AI must therefore not be static.
- Representative: To avoid bias, data should cover a variety of scenarios and demographic groups. A lack of diversity can make AI less effective. For example, a speech recognition model trained primarily on voices from a certain region may perform poorly with different accents.
- Security and confidentiality: It is imperative to ensure that data used by the AI model complies with current regulations, such as the GDPR in Europe, in order to protect the privacy of individuals. Similarly, careful evaluation is essential before any data is shared with public AI models, to avoid sensitive data (such as on customers, or projects being developed) reaching the public domain. It is therefore imperative to anonymize data before using it to power AI.
By adhering to these principles, organizations can begin to develop more secure, trustworthy and efficient AI, capable of performing optimally in a variety of contexts and for all users.
Which data sources should be used to feed AI?
To train and feed an AI model, organizations can provide data from multiple different sources: internal data, from its own operations; external data, often accessible via open data portals, social networks or search engines; and partner data.
Internal data: specific but potentially limited
Internal data is data collected directly by an organization in the course of its day-to-day activities. It includes detailed information, including on customers, transactions, production and logistics operations. This data is extremely specific and relevant for internal applications, as it directly reflects the organization’s own operations and activities. However, these datasets can suffer from significant limitations, including a lack of diversity and the presence of biases specific to the organization’s environment, which can restrict the ability of AI models to apply it effectively in wider contexts.
External data: providing additional context
External data, such as demographic or economic information plays a crucial role, in AI training, compensating for the limitations of internal data. It is often published by central/local/federal government bodies or international institutions, research or statistical organizations. This data provides diversity and scope that internal data cannot deliver, enabling AI models to benefit from richer context and more varied perspectives.
The use of demographic or economic data from government sources enables organizations to refine their algorithms to better predict consumer behavior and analyze market trends.
Essentially, an effective AI strategy must include a judicious mix of internal and external data. The former provides the specific detail needed for targeted applications, while the latter offers the scale and diversity required for robust, adaptive models.
When it comes to providing external data to strengthen AI models, Opendatasoft’s Data Hub offers an invaluable resource. Our portal provides access to over 33,000 datasets, making it easy to enrich internal datasets with diverse external perspectives. By integrating data from various sectors via the Data Hub, organizations can improve the accuracy of their AI models. This helps them not only to overcome internal data biases, but also to produce more robust analysis and more reliable predictions, improving their decision-making and competitiveness.
Partner data: win-win sharing
Finally, organizations can draw on data from partners, which adds further context and delivers an end-to-end, ecosystem view. For example, local authorities can draw on data from mobility players, energy players or even local businesses to deepen the context of their models.
Sharing data between partners also encourages greater collaboration, innovation and the creation of new, high value-added uses for data. It should therefore be recognized as a key data source when training AI models.
Data portal: the ideal solution to feed AI models while guaranteeing data quality and timeliness
If using an open data portal like the Data Hub is useful for enriching your AI with external data, integrating an internal data portal is just as important. Properly structured, this provides a middle stage for all information, between data production and sharing with an AI model.
Particular capabilities of internal data portals are especially relevant to AI:
- Centralization and constant updating: Centralizing all of an organization’s data assets via a portal enables more efficient information management, making it easier to access and regularly update data. This process ensures that AI algorithms are always working with the most up-to-date information, reducing the risk of prediction errors.
- Deduplication and data governance: As well as centralizing data, the portal helps to de-duplicate it and ensure data governance, ensuring accuracy and reliability. This step is essential to prevent data quality problems that can distort AI results down the line.
- Secure sharing: Having a data portal also enables the secure and controlled sharing of data with AI models and algorithms. This ensures that all sensitive data remains protected and that its use complies with current regulations, such as the GDPR for the protection of personal data.
In short, internal data portals have an indispensable role to play in powering AI models. They not only provide the necessary data, but also ensure that this data is accurate, up-to-date, diverse, and used ethically and compliantly.
AI and data portals: a clear fit
The synergy between data portals and artificial intelligence is essential to gaining the benefits of algorithms. As mathematician Cédric Villani pointed out in 2018, Data is the raw material of AI and the emergence of new uses and applications depends on it.” This underlines the crucial importance of effective data management and governance to optimizing the effectiveness of AI models.
Data portals, whether in-house or open, provide the necessary infrastructure to centralize, update and secure data, ensuring accurate predictions and well-informed decisions. It’s a combination of technology and information management that is essential to delivering the full benefits of artificial intelligence.
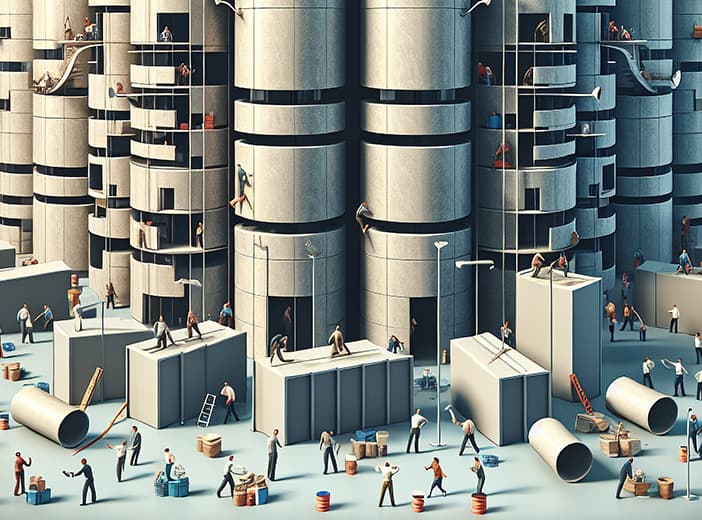
Organizational silos prevent data sharing and collaboration, increasing risk and reducing efficiency and innovation. How can companies remove them and ensure that data flows seamlessly around the organization so that it can be used by every employee?