Glossary
Data Mart
A subset of a data warehouse, a data mart is a way of storing data focused on a particular office, department, line of business area or subject.
What is a Data Mart?
A subset of a data warehouse, a data mart is a way of storing data focused on a particular office, department, line of business area (such as finance or marketing) or subject. It enables a defined group of users to quickly access relevant data, rather than having to search through the company’s entire data warehouse or other data sources.
A data mart is structured in the same way as a data warehouse, using Extract, Transform, Load (ETL) tools to add data and business intelligence tools to analyze information. Data marts can be:
- Dependent, with data coming solely from a central data warehouse
- Independent, collecting data from sources directly
- Hybrid, collecting information both from a data warehouse and from additional sources
How is a Data Mart different from a Data Warehouse or Data Lake?
A data mart is essentially a small section of a data warehouse, with the main difference being the amount (volume) and type of data it contains. While a data warehouse aims to centralize all of a company’s data across multiple subject areas via a structured model, a data mart is focused on a single subject area (such as a department). Data comes from the central data warehouse, data lake or additional sources. This means it contains less data and is therefore more agile and better performing when it comes to handling user queries.
A data lake differs from a data mart and data warehouse as it stores data in an unstructured way, without it being cleansed and processed. A data lake can feed a data mart, with the data mart adding structure to data as it is loaded.
What are the benefits of a Data Mart?
Data marts were introduced as many companies struggled with the size, complexity, and performance of their enterprise data warehouses. Easier to create and manage, data marts provide specific users with fast access to the focused data they need to do their jobs.
Data marts have four key benefits:
They are cheaper to create and manage
As they are smaller and less complex than a data warehouse or data lake, data marts are easier to create and less expensive to build and manage/maintain.
Faster access to specific users
Data marts contain less information, all of which is relevant to users. It is therefore easier and quicker for users to find the data they need, speeding up the creation of reports or dashboards. Data access can be given on a more granular user level, improving data governance and compliance.
Enables better decision-making
Data marts provide employees with easy access to the data they need to do their jobs. This leads to better, more data-driven decision making, positively impacting overall revenues. They act as a single source of truth for the department or area that they cover.
Better performance
As they contain less data (normally up to 100 GB), data marts tend to perform actions, such as running analysis, more quickly, speeding up access to data. Equally, managing and changing information is easier and less complex.
What are the challenges of Data Marts?
While they bring benefits, data marts also have three disadvantages when it comes to data management:
Data Marts share the same foundations as a data warehouse
As they are essentially smaller versions of a data warehouse, data marts share many of their challenges. They can be complex and expensive to manage and require modeling and data cleansing before data is made available to users.
Do not provide a comprehensive view
Data is focused on a specific use case or department. That means that users are unable to easily access data outside the data mart, even if it is stored in the wider data warehouse. This creates potential data silos within the organization, holding back data democratization.
Potentially lead to data inconsistencies
Enterprise data, such as from the data warehouse, may be shared and duplicated in multiple data marts – such as one for use by sales and one by marketing. That means that there is a risk that if data changes, these updates will not be applied across all data marts. This leads to inconsistencies and adds to management time and costs.
Learn more
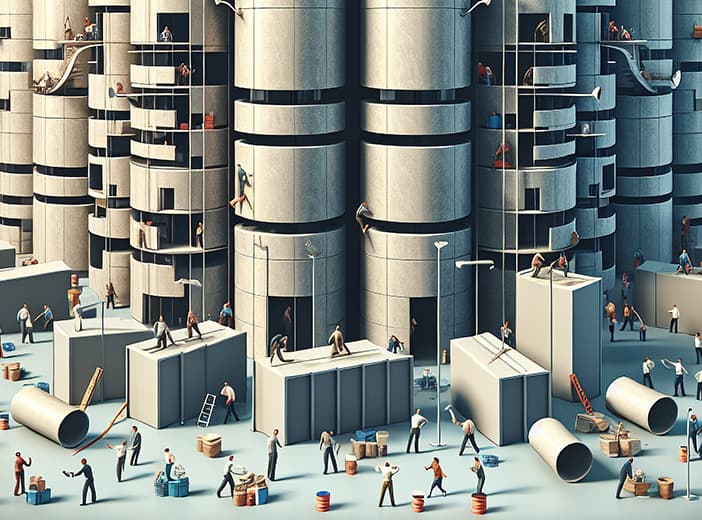
How to break down organizational silos to engage everyone in your data project
Organizational silos prevent data sharing and collaboration, increasing risk and reducing efficiency and innovation. How can companies remove them and ensure that data flows seamlessly around the organization so that it can be used by every employee?
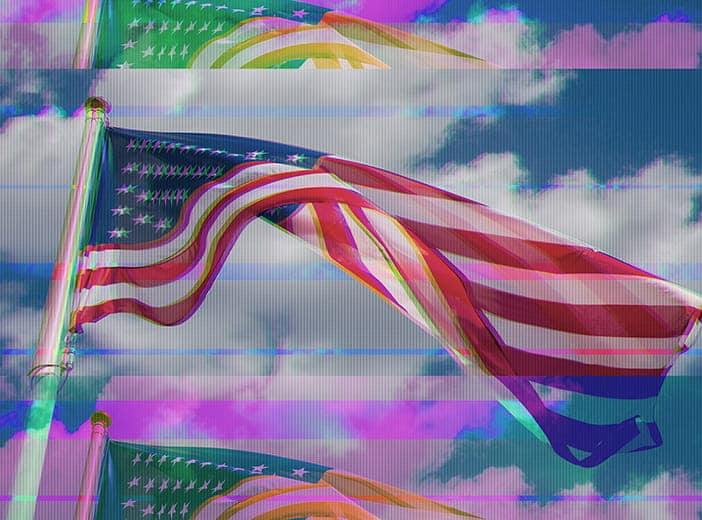
What the American Privacy Rights Act means for data governance
The American Privacy Rights Act (APRA) promises to increase the protection, privacy and security of consumer data, across the United States through federal legislation. We look at what it covers, next steps on its implementation and the benefits it brings to organizations as well as consumers.