3 Ways to Improve Data Quality in Your Organization
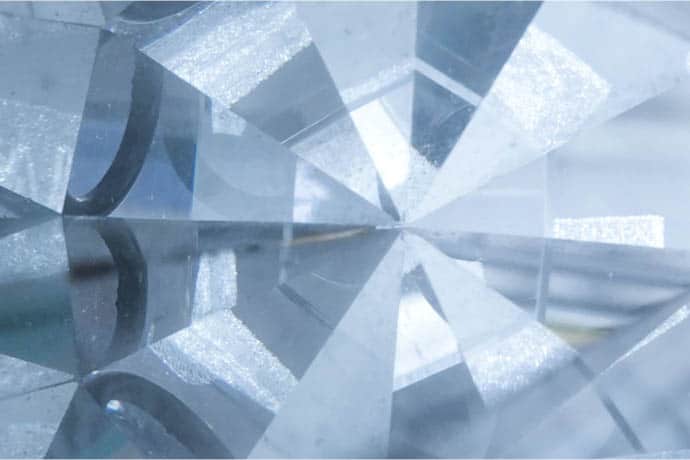
Low quality data affects us daily, often in ways we may not even notice. So, we need high quality data. But what is data quality and how do we get it?
Data helps us do amazing things. Whether it’s planning and building smarter cities or managing a crisis, data is a foundational element that enables us to work better. But the services we provide are only as good as the quality of the data they are built on. The old cliché of “garbage in, garbage out” definitely applies in the data world.
Low quality data can have a variety of consequences. A 2016 figure from IBM estimated that low quality data costs up to $3 trillion dollars per year in the US alone. Bad data quality can also lead us to overlook important events as they occur, to diagnose problems incorrectly, or even to prescribe the wrong solution to a pressing issue. Low quality data affects us daily, often in ways we may not even notice.
So, we need high quality data. But what is data quality and how do we get it?
What is data quality?
Measures of quality are all around us. Ranging from simple concepts like the familiar USDA Beef Quality Grades to more complex tools like the Air Quality Index (AQI), quality frameworks are designed to communicate information on how a specific item measures up against a trusted standard. Overall, quality frameworks help define what good looks like for a particular industry or issue.
Unfortunately for us, there is no universally agreed upon definition of data quality. However, there are terms that consistently appear in data quality discussions that can guide us in practice. Generally data is considered high quality if fits the intended purpose of its use. In addition, there are several dimensions commonly associated with high quality data.
- Accuracy – all data correctly reflects the object or event in the real world
- Completeness – all data that should be present is present
- Relevance – all data meets the requirements for intended use
- Timeliness – all data reflects the correct point in time
- Consistency – values and records are represented in the same way within/across datasets
There may be other dimensions added to these five like uniqueness, validity, or openness designed to capture different elements of the data that are important to particular users. But overall if your data meets the definitions of all or several of the dimensions noted above, it is high quality. Some organizations take it a step further and create their own data quality scores to help make the term more meaningful for their own users.
No matter how you define it, having good data quality is important. So how do we improve data quality in our organizations?
How do we improve data quality?
Improving data quality starts with understanding the data lifecycle.
A variety of factors including laws, systems, technology, training, and many others can affect data quality. Mapping data against the different stages of the lifecycle help us determine what quality issues we may be facing and what fixes may be appropriate.
No matter where your data is in the lifecycle, improving its quality is a long-term process. This work isn’t sexy, but it will pay off in the long run in better data, decisions, and outcomes. Following the three tips below can help you get started on your long-term journey to better data quality.
#1 – Describe your data well
Across all stages of the lifecycle, describing your data well is critical. As discussed in a previous blog, good description and metadata helps to provide context for data, standardizes formats and rules within and across organizations, and improves the use of data overall.
Good metadata improves the quality of data by improving consistency (one of the five dimensions mentioned above) and by creating a mechanism for starting to assess quality on the other four dimensions through the data lifecyle.
#2 – Prevent problems before they start
Correcting data errors is time consuming and difficult. Building in additional time for planning and preparation before you start collecting and analyzing data can help prevent errors from occurring and save valuable time and effort on the back end.
This work is often described as quality assurance and is essential work for a data governance or data management team. Good quality assurance work helps set goals for your data use, improves the relevance and timeliness of your data, and streamlines work at later stages in the data lifecyle.
#3 – Prioritize and correct common errors
No matter how much prevention you do, some errors will occur. Detecting and correcting errors, or quality control, is a key component of data quality. Quality control is often done manually but can be streamlined through the use of data profiling tools and by cataloging common data problems with simple fixes.
Using summary statistics to review your data can also help uncover potential errors that need correction. Correcting common errors helps improve the accuracy, completeness, and consistency of your data. Ensuring that people and resources are dedicated to this step is the last line of defense to improve data quality.
Putting high quality data to use
In the long run, high-quality data is a foundation. As data quality improves, your foundation gets stronger allowing more to be built on top of it and the potential uses of your data to multiply. Finding ways for your organization to prevent, detect, and correct data quality issues will set the stage for your data to be put into service in a variety of ways from improving mobility in your city to providing accurate information in a health crisis.
Stay tuned to the blog in the coming weeks and months to find out how to build on data quality with tools like real-time data sharing and APIs that can help you take the next steps in your data journey!
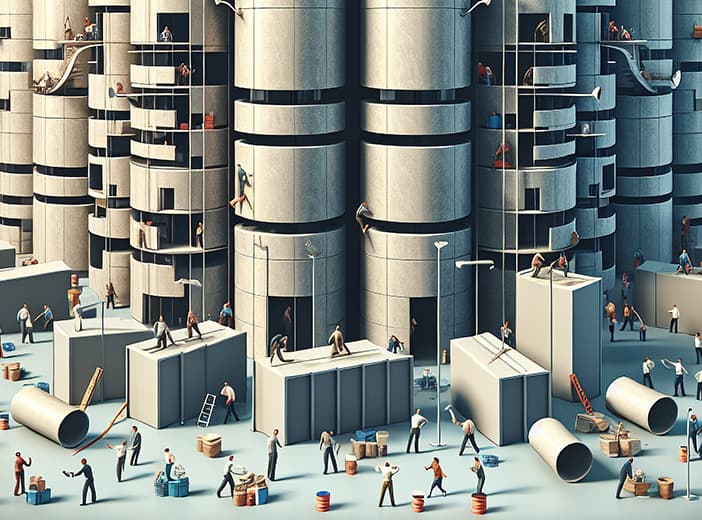
Organizational silos prevent data sharing and collaboration, increasing risk and reducing efficiency and innovation. How can companies remove them and ensure that data flows seamlessly around the organization so that it can be used by every employee?