Glossary
Data Pipeline
A data pipeline covers the steps involved in processing, optimizing and preparing raw data from disparate sources, so it can be used by the business
What is a Data Pipeline?
A data pipeline covers the steps involved in processing, optimizing and preparing raw data from disparate sources, so it can be used by the business. Through this workflow, data is taken from source systems, cleansed, transformed, enriched and delivered to its final destination, such as a data warehouse.
Modern data pipelines automate many of these steps to scale data management. Data pipelines normally use a variety of technologies, which collectively are referred to as the data stack.
Why are Data Pipelines important?
Organizations generate and collect increasing volumes of data from a wide range of systems. However, this raw data is useless – it needs to be cleansed, filtered, enriched and moved before it can be used effectively, such as for analysis. This is the role of a data pipeline, which eliminates manual steps, scaling data management for the organization. Using a data pipeline:
- Avoids data being lost or corrupted when it moves between systems, improving data quality
- Gives businesses control over their data, breaking down silos
- Increases understanding of data assets
- Improves efficiency through automation, freeing up staff time
- Enables data to be enriched to make it useful for the business, such as through analytics and Business Intelligence tools
- Underpins governance processes and mechanisms
- Allows companies to automate data management to drive greater efficiency
What are the steps in a Data Pipeline?
Data Pipelines combine multiple steps, which fall into three groups:
1. Data ingestion
Data is collected/extracted from source systems, via processes such as Extract, Transform, Load (ETL) and APIs.
2. Data Transformation
Data is then cleansed and processed to make it usable by the business. This could include steps to improve quality, to enrich it with additional data, and/or to combine it with other internal data sources.
3. Data Storage
Once transformed, data is then stored in a data repository (such as a data warehouse), where it is available to users. This is referred to as the data’s destination.
Successful data pipelines must be seamless, end-to-end and deliver high quality, trusted data. One of the key challenges to achieving this are dependencies. These are bottlenecks that require pipelines to wait (either for technical or business reasons) before dataflows can continue.
What are the types of Data Pipeline?
There are three main types of data pipeline:
Batch processing
Here batches of data are processed through the data pipeline at set time intervals. This is normally outside peak business hours to avoid impacting other computing workloads. Batch processing is the optimal solution when data is not required in real-time, such as accounting data used for month-end reporting.
Streaming data
This sees data continuously updated as it is created. For example, an event such as the sale of a product on an ecommerce website would automatically update the data stack, allowing real-time inventory management.
Lambda architecture
This hybrid approach combines batch and real-time processing in a single data pipeline. It is particularly useful in big data environments with different kinds of analytics applications.
What is the difference between Data Pipelines and Extract, Transform, Load (ETL)?
Extract, Transform and Load (ETL) is a key tool used within many data pipelines, but it is just a sub-process within the end-to-end pipeline. The main differences are:
- ETL pipelines follow a specific sequence (extract, transform, load). Data pipelines may follow different sequences of steps (such as extract, load, transform (ELT) used in data lakes)
- ETL pipelines tend to be used for batch processing, rather than stream processing in real-time
- Data pipelines do not always transform data – they may simply transport it to its destination (such as a data lake) where transformations are then applied
- Data pipelines are continuous and end-to-end, whereas ETL ends when data is loaded into the destination
Learn more
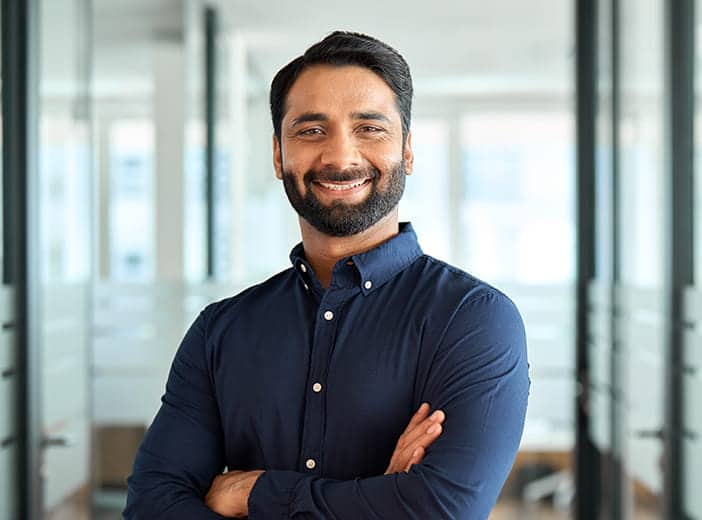
Overcoming the top 5 challenges faced by Chief Data Officers
Chief Data Officers are central to organizations becoming data-centric, maximizing data sharing to ensure that everyone has immediate access to the information they need. We explore the challenges they face - and how they can be overcome with the right strategy and technology.
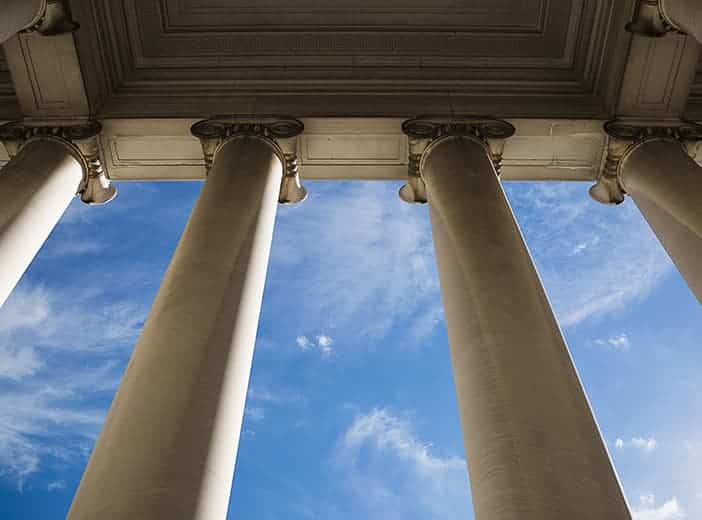
The importance of data exchanges to state-level collaboration
State governments cover an enormous range of services and are often complex, diverse organizations. Data sharing helps them become more collaborative, efficient and effective, building trust with stakeholders. How can data exchanges break down silos and enable data sharing and help them meet their key objectives?
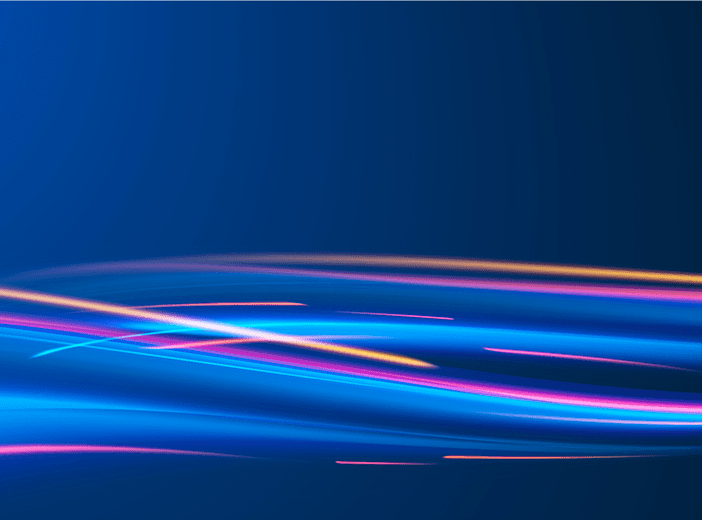
Everything you need to know about data enrichment
Data enrichment is essential to turning your raw data into valuable information that can be easily understood and used internally and externally. Our blog explains how to successfully implement data enrichment in your organization in order to improve quality, consistency, and standardization to maximize value.